Premier league betting analysis
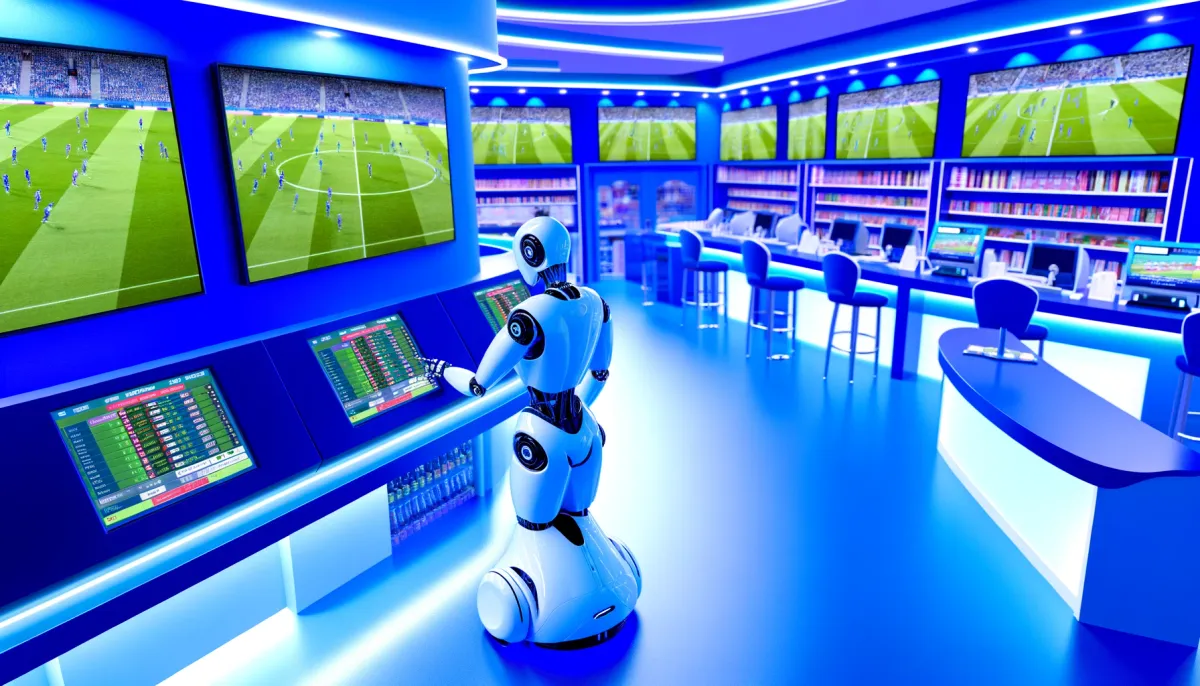
As the premiership season draws to a close for the 23/24 season, we revisit this article from 2 years ago. This article asked if backing any team with a level stake for each PL game could be profitable.
The analysis was performed using data sourced from https://www.football-data.co.uk and a set of Excel calculations. The analysis concluded that the most profitable teams were:
The five most profitable teams over 10 years with a level £1,000 stake.
- Crystal Palace (£68.4k)
- West Ham (£41.65k)
- Wolves (£37.16k)
- Tottenham (£33.59k)
- Burnley (£33.5k)
Two years later, we repeat the analysis, but now we have the power and ease of data analysis tools within ChatGPT 4o. We can now perform this analysis quicker and go much much deeper.
We downloaded the data for the last 10 seasons, added the format definition for each file and presented the archive to ChatGPT4o. The archive was decompressed and analysed without the need for a data pre-processing or formatting. This was impressive in itself and saved an hour of pre-processing compared with the previous attempt.
Below are the highlights from the ChatGPT4o session which indicates that there are still profitable teams to back, but there is a strategy that theoretically produces returns that are ten times better than the most successful team!
DISCLAIMER: This information is provided solely as a demonstration of the power of ChatGPT for data analysis using readily available data in a domain that is accessible for understanding. We do not advocate gambling and provide no warranties of any kind on the accuracy or future performance of the strategies described in this article.
Session Starts
The extracted files from the archive are:
notes.txt
PL-1415.csv
PL-1516.csv
PL-1617.csv
PL-1718.csv
PL-1819.csv
PL-1920.csv
PL-2021.csv
PL-2122.csv
PL-2223.csv
PL-2324.csv
To proceed, I will examine the contents of notes.txt
to understand any relevant context or instructions provided. Then, I will analyze the CSV files.
The notes.txt
file provides detailed information about the data contained in the CSV files. Here are the key points:
- The data is in CSV format and includes various statistics and betting odds related to football matches.
- There are abbreviations and terms used in the data, explained in the notes. Key terms include:
Div
: League DivisionDate
: Match Date (dd/mm/yy)Time
: Match kick-off timeHomeTeam
andAwayTeam
: Names of the home and away teamsFTHG
andFTAG
: Full Time Home/Away Team GoalsFTR
: Full Time Result (H=Home Win, D=Draw, A=Away Win)HTHG
andHTAG
: Half Time Home/Away Team GoalsHTR
: Half Time Result (H=Home Win, D=Draw, A=Away Win)- Detailed match statistics like attendance, referee, shots, corners, fouls, cards, and more.
The notes also mention various bookmakers and the type of betting odds included in the dataset.
Next, I will analyze the structure and content of one of the CSV files to understand the data better. Let's start with PL-1415.csv
.
The PL-1415.csv
file contains a variety of data points related to football matches. Here is a summary of the columns:
- Basic Match Information:
Div
: League DivisionDate
: Match DateHomeTeam
: Home TeamAwayTeam
: Away TeamFTHG
: Full Time Home Team GoalsFTAG
: Full Time Away Team GoalsFTR
: Full Time Result (H=Home Win, D=Draw, A=Away Win)HTHG
: Half Time Home Team GoalsHTAG
: Half Time Away Team GoalsHTR
: Half Time Result
- Betting Odds and Other Data:
- Columns like
Bb1X2
,BbMx>2.5
,BbAv<2.5
,BbAH
,BbAHh
,BbMxAHH
,BbAvAHH
,BbMxAHA
,BbAvAHA
,PSCH
,PSCD
,PSCA
represent various betting odds and bookmaker-related data.
- Columns like
There are 68 columns in total, which include a comprehensive set of data for each match.
Next, let's examine the overall structure of one more recent CSV file, such as PL-2324.csv
, to see if the structure and content have changed over time.
The PL-2324.csv
file, which contains more recent data, has expanded significantly in terms of columns. Here is a summary of the structure and some key points:
- Basic Match Information:
Div
: League DivisionDate
: Match DateTime
: Match kick-off timeHomeTeam
: Home TeamAwayTeam
: Away TeamFTHG
: Full Time Home Team GoalsFTAG
: Full Time Away Team GoalsFTR
: Full Time Result (H=Home Win, D=Draw, A=Away Win)HTHG
: Half Time Home Team GoalsHTAG
: Half Time Away Team GoalsHTR
: Half Time Result
- Additional Data:
- Various betting odds and statistics, which have significantly increased in number, indicating more detailed and comprehensive data over time.
The number of columns has increased from 68 in the PL-1415.csv
file to 106 in the PL-2324.csv
file, showing a greater inclusion of detailed betting odds and statistics.
To provide a thorough analysis, I will create a summary of key metrics and trends across these seasons. Please let me know if you have specific aspects or metrics you would like me to focus on.
Tim: Using this information calculate the team that would provide the largest returns given a level £1000 stake per game over the last 10 years using odds provided by Bet365.
ChatGPT: The team that would have provided the largest returns over the last 10 years, given a level £1000 stake per game, is Crystal Palace. The total return for betting on Crystal Palace is £49,170.
If you have any other specific analyses or insights you'd like to explore further, please let me know!
Tim: List the top 10 teams with the highest returns using the Bet365 odds
ChatGPT: The top 10 teams with the highest returns using Bet365 odds over the last 10 years, given a level £1000 stake per game, are:
- Crystal Palace: £49,170
- Swansea: £39,340
- Wolves: £34,000
- West Ham: £30,520
- Newcastle: £25,970
- Brentford: £17,530
- Leicester: £13,490
- Tottenham: £12,050
- Aston Villa: £7,430
- Cardiff: £7,150
If you need any further analysis or details, please let me know!
Tim: List the 10 teams with the largest losses using the Bet365 odds
ChatGPT: The 10 teams with the largest losses using Bet365 odds over the last 10 years, given a level £1000 stake per game, are:
- Southampton: -£52,910
- Chelsea: -£49,130
- Everton: -£44,740
- Brighton: -£38,010
- Norwich: -£33,170
- Sunderland: -£28,720
- Middlesbrough: -£26,170
- Fulham: -£24,020
- Sheffield United: -£21,320
- Huddersfield: -£21,090
If you need more information or further analysis, feel free to ask!
Tim: Break down the returns for the last 10 years for Crystal Palace using the Bet365 odds
ChatGPT: The annual returns for Crystal Palace using Bet365 odds over the last 10 years, given a level £1000 stake per game, are as follows:
- 2014/2015: £14,840
- 2015/2016: £380
- 2016/2017: £11,110
- 2017/2018: -£2,940
- 2018/2019: £20,910
- 2019/2020: -£1,950
- 2020/2021: £3,240
- 2021/2022: £9,050
- 2022/2023: -£9,340
- 2023/2024: £3,870
These values show the variation in returns across different seasons for Crystal Palace. If you need further analysis or additional details, please let me know!
Tim: Analyse the returns for backing the draw at £1000 per game using the Bet365 odds
ChatGPT: The annual returns for backing the draw at £1000 per game using Bet365 odds over the last 10 years are as follows:
- 2014/2015: -£28,470
- 2015/2016: £22,150
- 2016/2017: -£45,670
- 2017/2018: £18,840
- 2018/2019: -£98,490
- 2019/2020: -£23,370
- 2020/2021: -£46,690
- 2021/2022: -£33,320
- 2022/2023: -£34,970
- 2023/2024: -£43,860
These values show significant losses overall, indicating that backing the draw consistently has not been profitable over this period. If you need further analysis or have any other questions, please let me know!
Tim: What is the total return for backing the draw?
ChatGPT: The total return for backing the draw at £1000 per game using Bet365 odds over the last 10 years is -£313,850. This indicates a significant overall loss. If you have any further questions or need additional analysis, please let me know!
Tim: Calculate the returns for backing the home team and away team.
ChatGPT: Annual Returns for Backing the Home Team
- 2014/2015: -£7,320
- 2015/2016: -£33,670
- 2016/2017: £24,930
- 2017/2018: £10,980
- 2018/2019: £19,180
- 2019/2020: £16,010
- 2020/2021: -£62,680
- 2021/2022: -£39,370
- 2022/2023: £31,030
- 2023/2024: -£23,560
Annual Returns for Backing the Away Team
- 2014/2015: £600
- 2015/2016: £32,050
- 2016/2017: -£72,090
- 2017/2018: -£57,420
- 2018/2019: -£7,450
- 2019/2020: -£16,380
- 2020/2021: £108,760
- 2021/2022: -£16,890
- 2022/2023: -£61,950
- 2023/2024: -£52,100
Total Returns
- Home Team: The total return over the last 10 years is approximately -£84,470.
- Away Team: The total return over the last 10 years is approximately -£141,870.
If you need more insights or further analysis, please let me know!
Tim: Calculate the odds for backing the favourite verses the outsider.
ChatGPT: To calculate the returns for backing the favorite versus the outsider, we'll define the favorite as the team with lower odds for a given match, and the outsider as the team with higher odds. We'll then calculate the returns for backing the favorite and the outsider over the same period.
Steps:
- For each match, identify the favorite and the outsider based on the odds.
- Calculate the returns for backing the favorite and the outsider separately.
- Aggregate the returns over the entire period.
Let's proceed with this analysis.
Annual Returns for Backing the Favorite
- 2014/2015: -£9,610
- 2015/2016: -£41,660
- 2016/2017: £30,590
- 2017/2018: -£14,030
- 2018/2019: £1,100
- 2019/2020: -£34,040
- 2020/2021: -£30,570
- 2021/2022: £9,420
- 2022/2023: -£590
- 2023/2024: £7,780
Annual Returns for Backing the Outsider
- 2014/2015: £2,890
- 2015/2016: £40,040
- 2016/2017: -£77,750
- 2017/2018: -£32,410
- 2018/2019: £10,630
- 2019/2020: £33,670
- 2020/2021: £76,650
- 2021/2022: -£65,680
- 2022/2023: -£30,330
- 2023/2024: -£83,440
Total Returns
- Favorite: The total return over the last 10 years is approximately -£82,409.
- Outsider: The total return over the last 10 years is approximately -£126,730.
This analysis shows that backing the favorite generally resulted in smaller losses compared to backing the outsider over the past decade.
If you need more insights or further analysis, please let me know!
Tim: Calculate the returns based on each category of odds, grouping the odds into 10 categories.
ChatGPT: To calculate the returns based on categories of odds, we'll group the odds into 10 categories and calculate the returns for each category. Here are the steps:
- Define 10 categories of odds.
- Assign each match to one of the categories based on the odds.
- Calculate the returns for each category over the entire period.
Steps:
- Define the odds categories.
- For each match, assign the home, draw, and away odds to their respective categories.
- Calculate the returns for each odds category.
Let's proceed with this analysis.
Returns by Odds Category
The returns for backing each category of odds over the last 10 years, given a level £1000 stake per game, are as follows:
- 1.0-1.5: £0
- 1.5-2.0: -£24,890
- 2.0-2.5: £22,720
- 2.5-3.0: -£107,700
- 3.0-3.5: -£65,310
- 3.5-4.0: -£12,230
- 4.0-4.5: -£124,320
- 4.5-5.0: -£89,810
- 5.0-5.5: -£54,900
- 5.5-6.0: £20,500
- 6.0-6.5: £3,750
- 6.5-7.0: -£41,250
- 7.0-7.5: -£750
- 7.5-8.0: -£10,000
- 8.0-8.5: -£57,000
- 8.5-9.0: £7,000
- 9.0-9.5: £10,500
- 9.5-10.0: £4,000
- >10.5: -£4,000
These values show the returns for backing each odds category. As observed, certain categories, particularly those with lower odds, generally resulted in lower returns or losses, while some categories with higher odds also faced significant losses. A few categories provided positive returns.
If you need further insights or additional analysis, please let me know!
Tim: Do these values show a correlation between positive returns and odds offered?
ChatGPT: To determine if there is a correlation between positive returns and the odds offered, we can calculate the correlation coefficient between the categories of odds and their corresponding returns. This will give us a numerical value indicating the strength and direction of the correlation.
Steps:
- Calculate the correlation coefficient between odds categories and returns.
- Visualize the relationship using a scatter plot.
Let's proceed with this analysis.
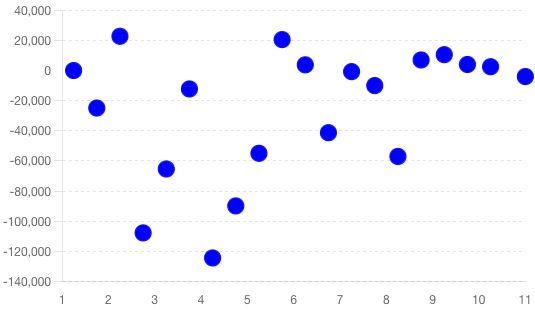
The correlation coefficient between the mid-points of the odds categories and their corresponding returns is approximately 0.339. This value indicates a moderate positive correlation, suggesting that there is a tendency for higher odds to be associated with higher returns (or smaller losses).
The scatter plot visually supports this, showing a general trend where higher odds categories (mid-points) often correspond to higher returns, though the relationship is not strong and there is considerable variability.
Interpretation:
- A correlation coefficient of 0.339 indicates a moderate positive relationship, meaning that as the odds increase, the returns tend to increase as well, but not consistently.
- The variability in returns across different odds categories suggests that other factors may also play a significant role in determining returns.
If you need more detailed analysis or further insights, please let me know!
Tim: Analyse the relationship between the day of the week and the returns for the favourite. For example, do the favourites do better on Saturday matches versus mid week matches.
ChatGPT: To analyze the relationship between the day of the week and the returns for the favorite, we will:
- Extract the day of the week for each match.
- Categorize each match as either a favorite win or loss based on the odds.
- Calculate the returns for backing the favorite on each day of the week.
- Compare the returns for each day.
Let's proceed with this analysis.
It seems there are some date entries that are not properly formatted or are missing, causing the pd.to_datetime
function to fail. We need to handle these cases and ensure all dates are properly converted.
Let's update the function to handle such cases gracefully.
Returns for Favorites by Day of the Week
The returns for backing the favorite, given a level £1000 stake per game, are as follows:
- Monday: £3,340
- Tuesday: -£3,980
- Wednesday: £15,950
- Thursday: -£8,930
- Friday: £690
- Saturday: £13,950
- Sunday: £960
Interpretation
- Positive Returns: Wednesday and Saturday have the highest positive returns, indicating that favorites tend to perform better on these days.
- Negative Returns: Thursday and Tuesday show significant negative returns, suggesting that favorites tend to underperform on these days.
If you need further insights or additional analysis, please let me know!
Tim: Repeat for the outsiders
ChatGPT: Returns for Outsiders by Day of the Week
The returns for backing the outsider, given a level £1000 stake per game, are as follows:
- Monday: -£3,600
- Tuesday: £14,980
- Wednesday: -£44,000
- Thursday: -£700
- Friday: £4,100
- Saturday: -£22,820
- Sunday: -£21,820
Interpretation
- Positive Returns: Tuesday and Friday show positive returns, indicating that outsiders tend to perform better on these days.
- Negative Returns: Wednesday, Saturday, and Sunday show significant negative returns, suggesting that outsiders tend to underperform on these days.
If you need further insights or additional analysis, please let me know!
Tim: Calculate the returns when backing teams that suffered a large loss in the previous game.
ChatGPT: To calculate the returns when backing teams that suffered a large loss in their previous game, we need to:
- Define what constitutes a "large loss."
- Track the results of teams across multiple games.
- Identify games where a team suffered a large loss in the previous game.
- Calculate the returns for betting on these teams in their next game.
Steps:
- Define a "large loss" (e.g., losing by 3 or more goals).
- Track the performance of teams across games in each season.
- Identify instances of large losses and the subsequent games.
- Calculate the returns for backing these teams in their next game.
Let's proceed with this analysis.
The total return for backing teams that suffered a large loss (defined as losing by 3 or more goals) in their previous game, given a level £1000 stake per game, is -£97,550.
This significant negative return suggests that backing teams to bounce back after a large loss has not been a profitable strategy over the last 10 years. If you need further insights or additional analysis, please let me know!
Tim: Generate other betting strategies based on this data that could prove profitable
ChatGPT: To identify potentially profitable betting strategies based on the provided data, we can explore several approaches, including:
- Momentum Betting: Backing teams that have won multiple consecutive games.
- Form Reversal: Betting on teams that have had a string of losses to win.
- Undervalued Odds: Identifying and backing teams with odds that appear undervalued based on their recent performance.
- Home/Away Performance: Betting on teams with strong home or away performance.
- Goals-Based Strategies: Betting based on over/under total goals.
Tim: They sound very interesting, but we will save analysis of those for another day!
Comments ()