Causal AI
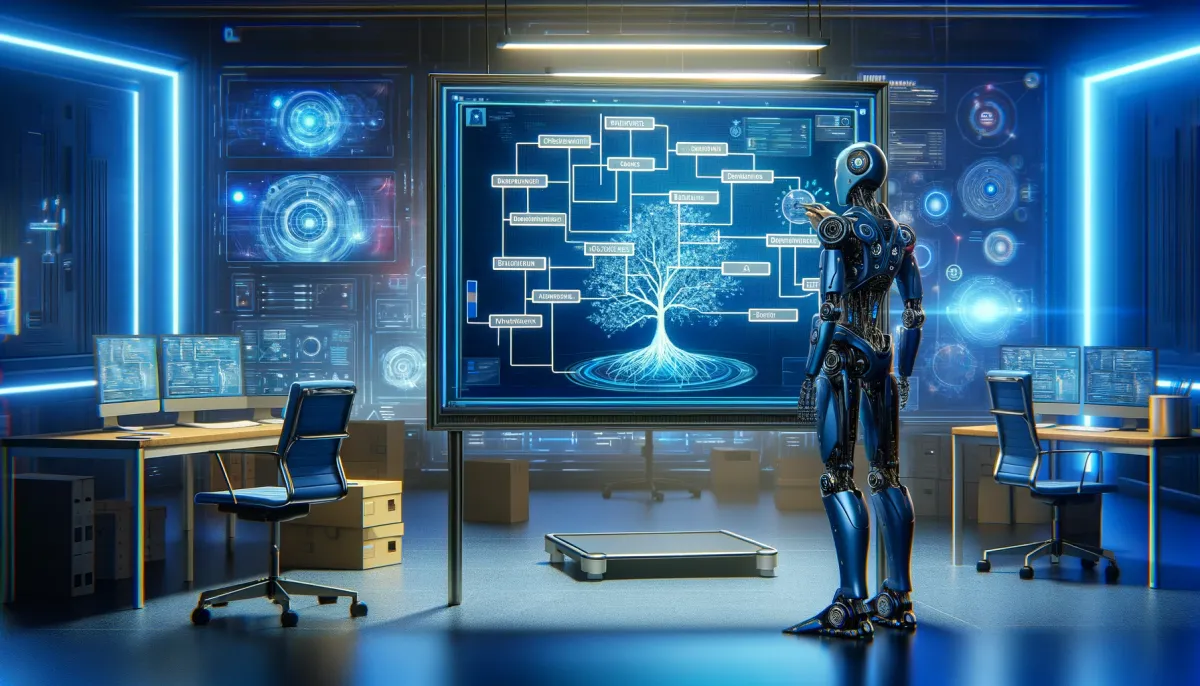
Tim: Let's have a conversation about Causal AI. Keep your answers to less than a paragraph and conversational and finish each answer with a question back to me.
ChatGPT: Oh, Causal AI! That's a fascinating area, focusing on understanding the cause-and-effect relationships in data, rather than just correlations. This can lead to more accurate predictions and insights. What aspects of causal AI are you most interested in?
Tim: What are the differences between Causal AI and Non-causal AI?
ChatGPT: The key difference between Causal AI and Non-causal AI lies in how they handle data and relationships. Non-causal AI, like most traditional machine learning, identifies patterns and correlations in data. It's great for prediction when the environment doesn't change much. Causal AI, on the other hand, tries to understand the cause-and-effect relationships behind the data. This allows it to make predictions and decisions even when the environment changes, making it potentially more robust and insightful. Do you think understanding causality is crucial for the future of AI?
Tim: Yes. I think for us to feel confident in adopting AI, we have to be able to explain the actions or recommendations that are being made. If Causal AI allows for environmental changes and is more robust and insightful, that sound like a good thing. Does Causal AI have any disadvantages over the more traditional AI techniques?
ChatGPT: You're right in recognising the importance of explainability in AI adoption. Causal AI does have some disadvantages compared to traditional AI techniques. One key challenge is the complexity in modeling causal relationships, especially in situations where the causal factors are not well understood or are numerous. Also, obtaining data that can effectively inform causal inferences can be more difficult and expensive. Finally, causal models can sometimes be less accurate in predictions compared to traditional correlation-based models, especially in tightly controlled environments where external factors are minimal. Do you think these challenges are surmountable in the near future?
Tim: It sounds like humans would still be responsible for doing the initial modelling, identifying possible causal relationships and presenting data to support these models. I could see how this could be challenging in some cases. Are there any real-life use cases where Causal AI is already being used?
ChatGPT: Yes, humans play a crucial role in framing causal models and hypotheses. Real-life use cases of Causal AI are emerging across various sectors. In healthcare, it's being used to understand the causal pathways of diseases and the impact of treatments. In economics, it helps in understanding the causal impact of policy changes or economic interventions. Marketing is another field where it's applied to understand the causal effect of advertising campaigns on consumer behavior. Additionally, in the realm of social media, it's used to understand the causal effects of content on user engagement and behavior. Are there any specific sectors where you think Causal AI could be particularly beneficial?
Tim: Of the list you mentioned, I think Healthcare sounds like a big opportunity. If we can understand which treatment was actually effective when a range of treatments are being used, that sounds like it could save lives, time and money. From a compliance perspective, I am interested in the cause and effect of unethical behaviour within large organisations. During a compliance investigation, it might be interesting to explore what factors led up to a compliance or regulatory breach so that policies and procedures could be updated to prevent a future reoccurrence. What do you think about applications in the compliance and risk domain?
ChatGPT: The compliance and risk domain is indeed a promising area for the application of Causal AI. Understanding the root causes of compliance breaches or unethical behavior in large organizations can lead to more effective preventive measures. Causal AI could analyze complex data from various sources within an organization to identify patterns and factors leading to such incidents. This insight could be invaluable in updating policies and procedures, as well as in training programs to mitigate future risks. Additionally, it could help in proactive risk management by predicting potential areas of concern before they manifest into bigger issues. How do you think organisations might balance the use of such AI tools with concerns about employee privacy and data security?
Tim: Compliance teams themselves should have policies and procedures that guide what data can be used to train AI systems. The use of personally identifiable or proprietary information should be avoided. Audit teams should provide oversight to ensure that compliance teams are following their own policies and procedures. If the AI models are looking for patterns, then perhaps the data could be anonymised until a potential breach is found. Can AI help detect whether there is private or proprietary data within a data set?
ChatGPT: Yes, AI can certainly assist in detecting private or proprietary data within datasets. There are AI tools specifically designed for data governance and privacy compliance. These tools can scan through datasets to identify and flag sensitive information, such as personal identifiers or confidential business information. They use algorithms to recognize patterns and markers that signify sensitive data. Additionally, AI can assist in anonymizing data by removing or obfuscating identifiable information, making it safer for analysis while preserving its usefulness. This way, compliance teams can leverage AI for pattern detection and causal analysis without compromising privacy or security. In your opinion, would the implementation of such AI tools be widely accepted in your industry?
Tim: People need to see to believe, if the AI tools are transparent and can be explained then adoption will increase. Which companies are promoting and producing Causal AI applications?
ChatGPT: The adoption of AI tools indeed often hinges on transparency and explainability. Several companies and startups are at the forefront of developing and promoting Causal AI applications:
- Microsoft: Through its research labs, Microsoft has been exploring causal inference in various contexts, including healthcare and economics.
- Google: Google has been incorporating causal reasoning in various projects, particularly in understanding user behavior and improving its algorithms.
- IBM: IBM has been investing in AI that can understand causality, particularly for applications in business and healthcare.
- DoWhy: It's an open-source project by Microsoft for causal inference that's gaining popularity among data scientists.
- CausalNex: A software library by QuantumBlack, a McKinsey company, focuses on Bayesian networks to model causal relationships.
- Pearl.ai: Named after Judea Pearl, a pioneer in causal inference, this company specializes in causal AI and its applications in different industries.
These companies are pushing the boundaries in Causal AI, aiming to make it more accessible and practical for a variety of uses. Do you think the involvement of these major players will accelerate the adoption of causal reasoning in AI across different industries?
Tim: I hope so. It sounds very promising! Thank you for the conversation.
ChatGPT: You're welcome! If you have any more questions in the future or need assistance with anything else, feel free to reach out. Have a great day!
Comments ()